Topic:Clustering Multivariate Time Series
What is Clustering Multivariate Time Series? Clustering multivariate time-series is the process of grouping similar time-series data with more than one timestamped variable based on their patterns and characteristics.
Papers and Code
Dec 29, 2024
Abstract:Distance measures have been recognized as one of the fundamental building blocks in time-series analysis tasks, e.g., querying, indexing, classification, clustering, anomaly detection, and similarity search. The vast proliferation of time-series data across a wide range of fields has increased the relevance of evaluating the effectiveness and efficiency of these distance measures. To provide a comprehensive view of this field, this work considers over 100 state-of-the-art distance measures, classified into 7 categories: lock-step measures, sliding measures, elastic measures, kernel measures, feature-based measures, model-based measures, and embedding measures. Beyond providing comprehensive mathematical frameworks, this work also delves into the distinctions and applications across these categories for both univariate and multivariate cases. By providing comprehensive collections and insights, this study paves the way for the future development of innovative time-series distance measures.
Via
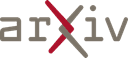
Dec 13, 2024
Abstract:Gravitational-wave observatories like LIGO are large-scale, terrestrial instruments housed in infrastructure that spans a multi-kilometer geographic area and which must be actively controlled to maintain operational stability for long observation periods. Despite exquisite seismic isolation, they remain susceptible to seismic noise and other terrestrial disturbances that can couple undesirable vibrations into the instrumental infrastructure, potentially leading to control instabilities or noise artifacts in the detector output. It is, therefore, critical to characterize the seismic state of these observatories to identify a set of temporal patterns that can inform the detector operators in day-to-day monitoring and diagnostics. On a day-to-day basis, the operators monitor several seismically relevant data streams to diagnose operational instabilities and sources of noise using some simple empirically-determined thresholds. It can be untenable for a human operator to monitor multiple data streams in this manual fashion and thus a distillation of these data-streams into a more human-friendly format is sought. In this paper, we present an end-to-end machine learning pipeline for features-based multivariate time series clustering to achieve this goal and to provide actionable insights to the detector operators by correlating found clusters with events of interest in the detector.
* 8 pages, 6 figures, Accepted to The 5th International Workshop on Big
Data & AI Tools, Methods, and Use Cases for Innovative Scientific Discovery
(BTSD 2024)
Via
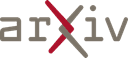
Dec 14, 2024
Abstract:Multivariate time series forecasting is crucial for various applications, such as financial investment, energy management, weather forecasting, and traffic optimization. However, accurate forecasting is challenging due to two main factors. First, real-world time series often show heterogeneous temporal patterns caused by distribution shifts over time. Second, correlations among channels are complex and intertwined, making it hard to model the interactions among channels precisely and flexibly. In this study, we address these challenges by proposing a general framework called \textbf{DUET}, which introduces \underline{DU}al clustering on the temporal and channel dimensions to \underline{E}nhance multivariate \underline{T}ime series forecasting. First, we design a Temporal Clustering Module (TCM) that clusters time series into fine-grained distributions to handle heterogeneous temporal patterns. For different distribution clusters, we design various pattern extractors to capture their intrinsic temporal patterns, thus modeling the heterogeneity. Second, we introduce a novel Channel-Soft-Clustering strategy and design a Channel Clustering Module (CCM), which captures the relationships among channels in the frequency domain through metric learning and applies sparsification to mitigate the adverse effects of noisy channels. Finally, DUET combines TCM and CCM to incorporate both the temporal and channel dimensions. Extensive experiments on 25 real-world datasets from 10 application domains, demonstrate the state-of-the-art performance of DUET.
* Accepted by KDD 2025
Via
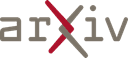
Nov 13, 2024
Abstract:Physical-Layer Authentication (PLA) offers endogenous security, lightweight implementation, and high reliability, making it a promising complement to upper-layer security methods in Edge Intelligence (EI)-empowered Industrial Internet of Things (IIoT). However, state-of-the-art Channel State Information (CSI)-based PLA schemes face challenges in recognizing mobile multi-users due to the limited reliability of CSI fingerprints in low Signal-to-Noise Ratio (SNR) environments and the constantly shifting CSI distributions with user movements. To address these issues, we propose a Temporal Dynamic Graph Convolutional Network (TDGCN)-based PLA scheme. This scheme harnesses Intelligent Reflecting Surfaces (IRSs) to refine CSI fingerprint precision and employs Graph Neural Networks (GNNs) to capture the spatio-temporal dynamics induced by user movements and IRS deployments. Specifically, we partition hierarchical CSI fingerprints into multivariate time series and utilize dynamic GNNs to capture their associations. Additionally, Temporal Convolutional Networks (TCNs) handle temporal dependencies within each CSI fingerprint dimension. Dynamic Graph Isomorphism Networks (GINs) and cascade node clustering pooling further enable efficient information aggregation and reduced computational complexity. Simulations demonstrate the proposed scheme's superior authentication accuracy compared to seven baseline schemes.
* 9 pages, 12 figures
Via
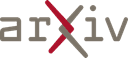
Oct 20, 2024
Abstract:This paper addresses two tasks: (i) fixed-size objects such as hay bales are to be identified in an aerial image for a given reference image of the object, and (ii) variable-size patches such as areas on fields requiring spot spraying or other handling are to be identified in an image for a given small-scale reference image. Both tasks are related. The second differs in that identified sub-images similar to the reference image are further clustered before patches contours are determined by solving a traveling salesman problem. Both tasks are complex in that the exact number of similar sub-images is not known a priori. The main discussion of this paper is presentation of an acceleration mechanism for sub-image search that is based on a transformation of an image to multivariate time series along the RGB-channels and subsequent segmentation to reduce the 2D search space in the image. Two variations of the acceleration mechanism are compared to exhaustive search on diverse synthetic and real-world images. Quantitatively, proposed method results in solve time reductions of up to 2 orders of magnitude, while qualitatively delivering comparative visual results. Proposed method is neural network-free and does not use any image pre-processing.
* 10 pages, 9 figures, 3 tables
Via
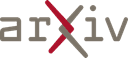
Oct 16, 2024
Abstract:Anomaly detection in multivariate time series is challenging as heterogeneous subsequence anomalies may occur. Reconstruction-based methods, which focus on learning nomral patterns in the frequency domain to detect diverse abnormal subsequences, achieve promising resutls, while still falling short on capturing fine-grained frequency characteristics and channel correlations. To contend with the limitations, we introduce CATCH, a framework based on frequency patching. We propose to patchify the frequency domain into frequency bands, which enhances its ability to capture fine-grained frequency characteristics. To perceive appropriate channel correlations, we propose a Channel Fusion Module (CFM), which features a patch-wise mask generator and a masked-attention mechanism. Driven by a bi-level multi-objective optimization algorithm, the CFM is encouraged to iteratively discover appropriate patch-wise channel correlations, and to cluster relevant channels while isolating adverse effects from irrelevant channels. Extensive experiments on 9 real-world datasets and 12 synthetic datasets demonstrate that CATCH achieves state-of-the-art performance.
Via
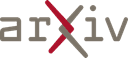
Sep 26, 2024
Abstract:The application of Shapley values to high-dimensional, time-series-like data is computationally challenging - and sometimes impossible. For $N$ inputs the problem is $2^N$ hard. In image processing, clusters of pixels, referred to as superpixels, are used to streamline computations. This research presents an efficient solution for time-seres-like data that adapts the idea of superpixels for Shapley value computation. Motivated by a forensic DNA classification example, the method is applied to multivariate time-series-like data whose features have been classified by a convolutional neural network (CNN). In DNA processing, it is important to identify alleles from the background noise created by DNA extraction and processing. A single DNA profile has $31,200$ scan points to classify, and the classification decisions must be defensible in a court of law. This means that classification is routinely performed by human readers - a monumental and time consuming process. The application of a CNN with fast computation of meaningful Shapley values provides a potential alternative to the classification. This research demonstrates the realistic, accurate and fast computation of Shapley values for this massive task
* 16 pages, 5 figures
Via
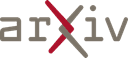
Jul 14, 2024
Abstract:We introduce an anomaly detection method for multivariate time series data with the aim of identifying critical periods and features influencing extreme climate events like snowmelt in the Arctic. This method leverages the Variational Autoencoder (VAE) integrated with dynamic thresholding and correlation-based feature clustering. This framework enhances the VAE's ability to identify localized dependencies and learn the temporal relationships in climate data, thereby improving the detection of anomalies as demonstrated by its higher F1-score on benchmark datasets. The study's main contributions include the development of a robust anomaly detection method, improving feature representation within VAEs through clustering, and creating a dynamic threshold algorithm for localized anomaly detection. This method offers explainability of climate anomalies across different regions.
* This work was presented at the 2024 IEEE International Geoscience and
Remote Sensing Symposium, IGARSS 2024, 07-12 July 2024, Athens, Greece
Via
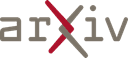
Jul 16, 2024
Abstract:We propose a deep generative approach using latent temporal processes for modeling and holistically analyzing complex disease trajectories, with a particular focus on Systemic Sclerosis (SSc). We aim to learn temporal latent representations of the underlying generative process that explain the observed patient disease trajectories in an interpretable and comprehensive way. To enhance the interpretability of these latent temporal processes, we develop a semi-supervised approach for disentangling the latent space using established medical knowledge. By combining the generative approach with medical definitions of different characteristics of SSc, we facilitate the discovery of new aspects of the disease. We show that the learned temporal latent processes can be utilized for further data analysis and clinical hypothesis testing, including finding similar patients and clustering SSc patient trajectories into novel sub-types. Moreover, our method enables personalized online monitoring and prediction of multivariate time series with uncertainty quantification.
* Accepted at Machine Learning for Healthcare 2024. arXiv admin note:
substantial text overlap with arXiv:2311.08149
Via
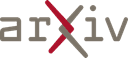
May 14, 2024
Abstract:Multivariate time series forecasting tasks are usually conducted in a channel-dependent (CD) way since it can incorporate more variable-relevant information. However, it may also involve a lot of irrelevant variables, and this even leads to worse performance than the channel-independent (CI) strategy. This paper combines the strengths of both strategies and proposes the Deep Graph Clustering Transformer (DGCformer) for multivariate time series forecasting. Specifically, it first groups these relevant variables by a graph convolutional network integrated with an autoencoder, and a former-latter masked self-attention mechanism is then considered with the CD strategy being applied to each group of variables while the CI one for different groups. Extensive experimental results on eight datasets demonstrate the superiority of our method against state-of-the-art models, and our code will be publicly available upon acceptance.
Via
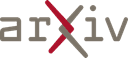